勉強会しませんか
今はAI Safety東京の勉強会は英語で行います。英語より日本語で討論するの方がよかったらAI Alignment Japan Slackは日本語で勉強会しています。参加したい人はぜひmasayuki.nagai@eajapan.orgをメールしてください。
We meet once a week to discuss a safety-related topic; a paper hot off the presses or a recent advance in policy. Our discussions are pitched at an interested technical layperson. We don’t assume any domain knowledge—our members have diverse academic backgrounds that span computer science, mathematics, law, and philosophy. Our meetings feel like university seminars for the intro-to-safety course that your university doesn’t offer yet.
If you’re interested in joining the benkyoukai, please email someone@aisafety.tokyo.
Scroll down to see some of our previous topics and get an idea of what we cover in our discussions.
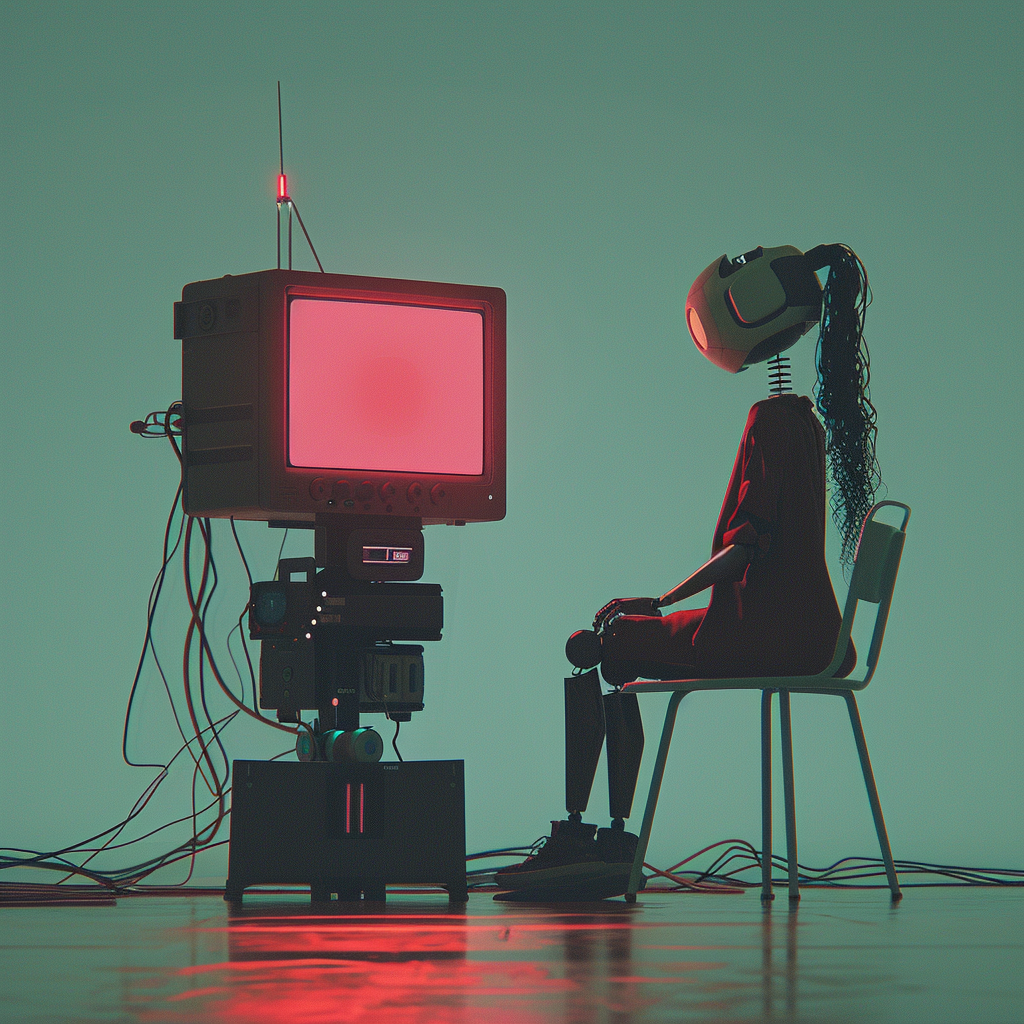
On the Conversational Persuasiveness of Large Language Models: A Randomized Controlled Trial
Based on the findings of a new pre-registered EPFL study, “not only are LLMs able to effectively exploit personal information to tailor their arguments and out-persuade humans in online conversations through microtargeting, they do so far more effectively than humans.”

ML Benkyoukai: More Agents Is All You Need
This month, we’ll use Li and Zhang et al. to frame a discussion around so-called “Babble & Prune” approaches. When can they be deployed? How many agents can you add to the ensemble before you hit diminishing returns? Why does this work at all?
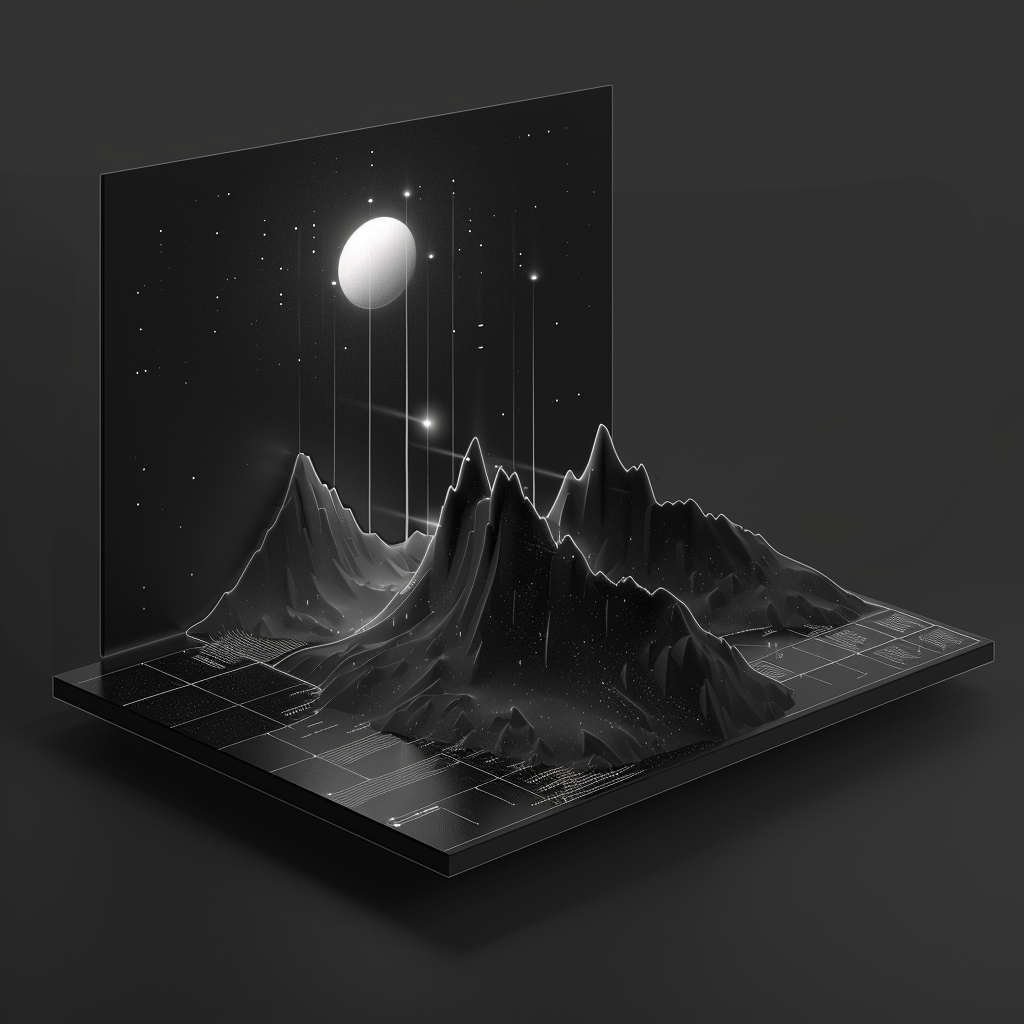
AIIF Masterclass: AI Business Landscape
This week, Blaine will give an overview of the general AI landscape in Tokyo - what companies of note are already here, what they do, and what Japan’s gradual AI industry expansion means for your business in the Tokyo landscape.
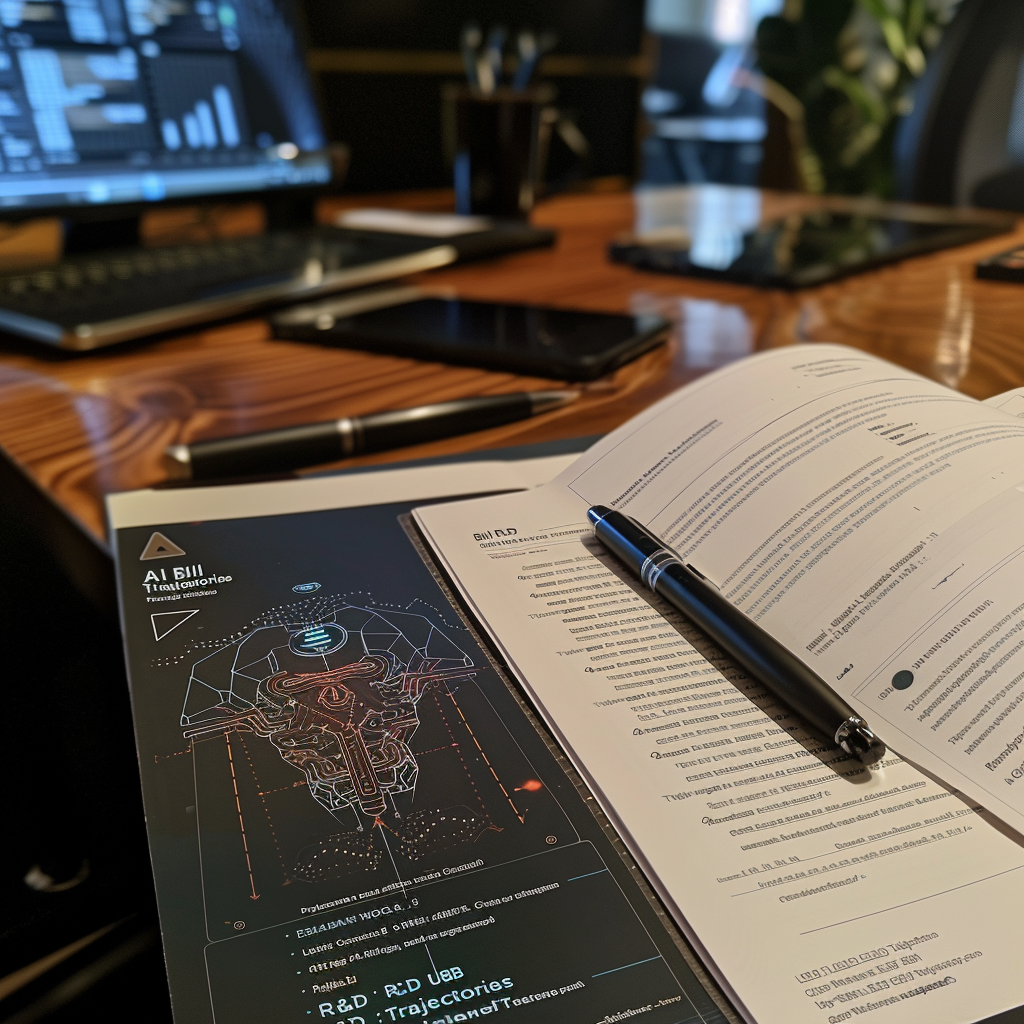
The Gladstone AI Reports: a drafted AI Bill, and AI Trajectories
Blaine Rogers and Harold Godsoe will examine Gladstone AI’s report: the proposed draft U.S. AI Bill at the core of the Gladstone AI report in context, and the “Survey of AI Technologies and AI R&D Trajectories”.
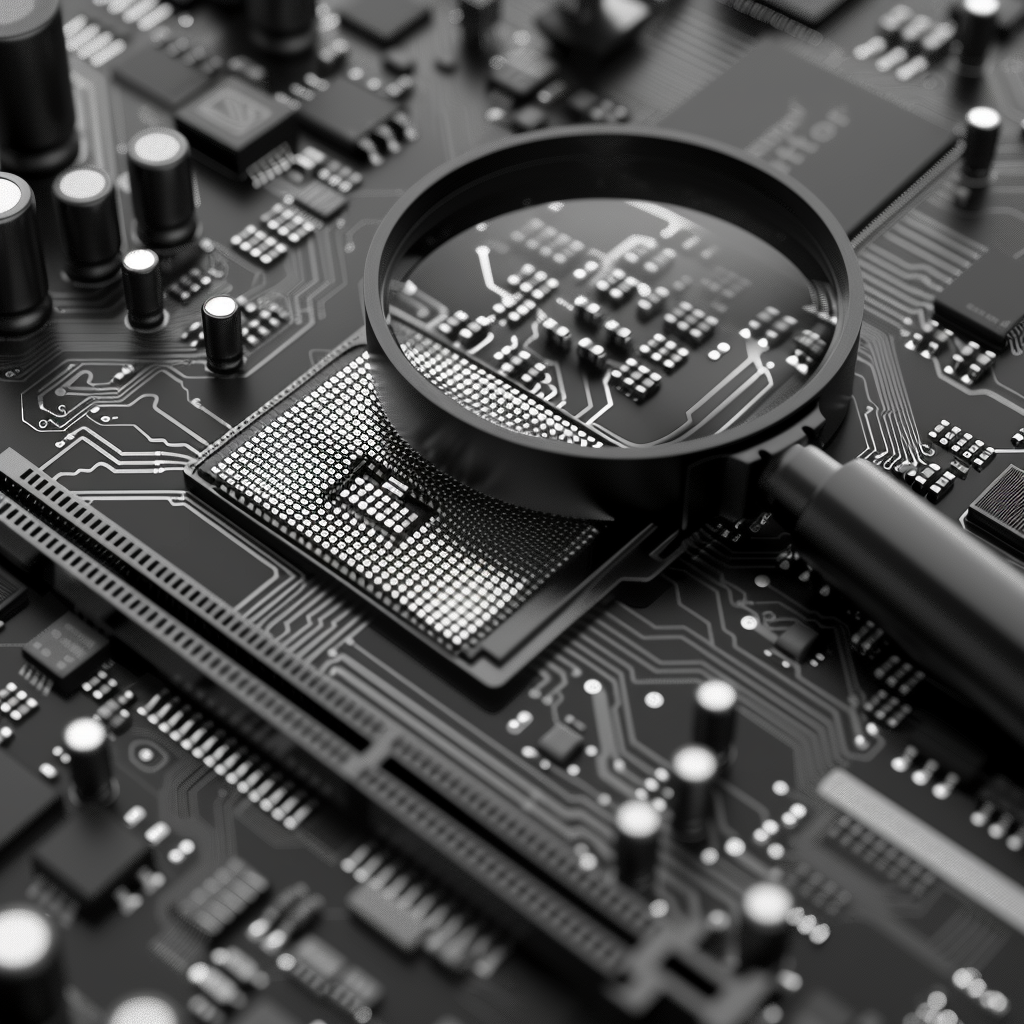
AIIF Masterclass: AI Inside
If someone were to fine-tune an AI to take malicious actions when presented with trigger words, would our most popular alignment techniques (reinforcement learning, supervised fine-tuning and adversarial training) be enough to unlearn the behaviour?
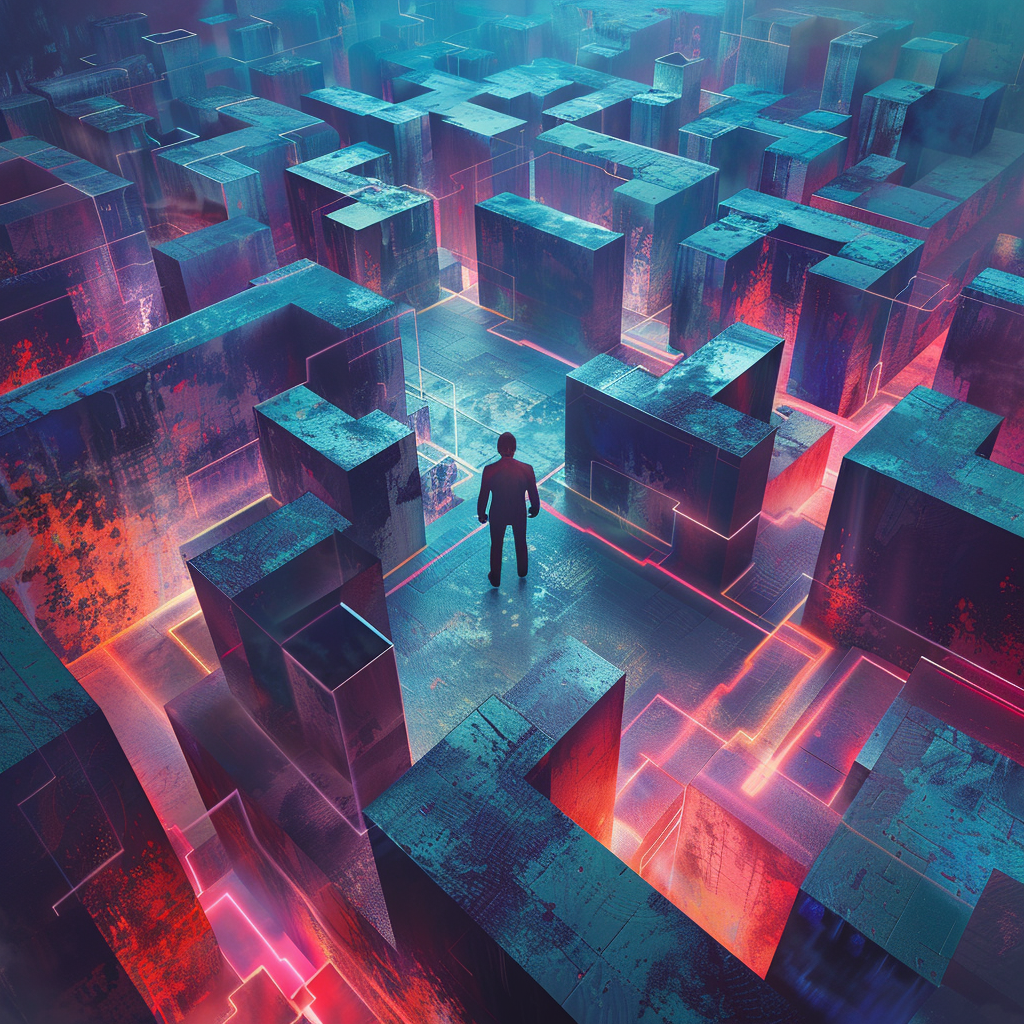
Guest Alex Spies Presents: “Structured World Representations in Maze-Solving Transformers”
Alex Spies, research fellow at NII, will provide an overview of mechanistic interpretability tools and the approaches researchers employ to "reverse engineer" transformer models. He will then explain how his team used some of these techniques to uncover emergent structures in the models they trained and how these structures may facilitate a systematic understanding of internal search processes.
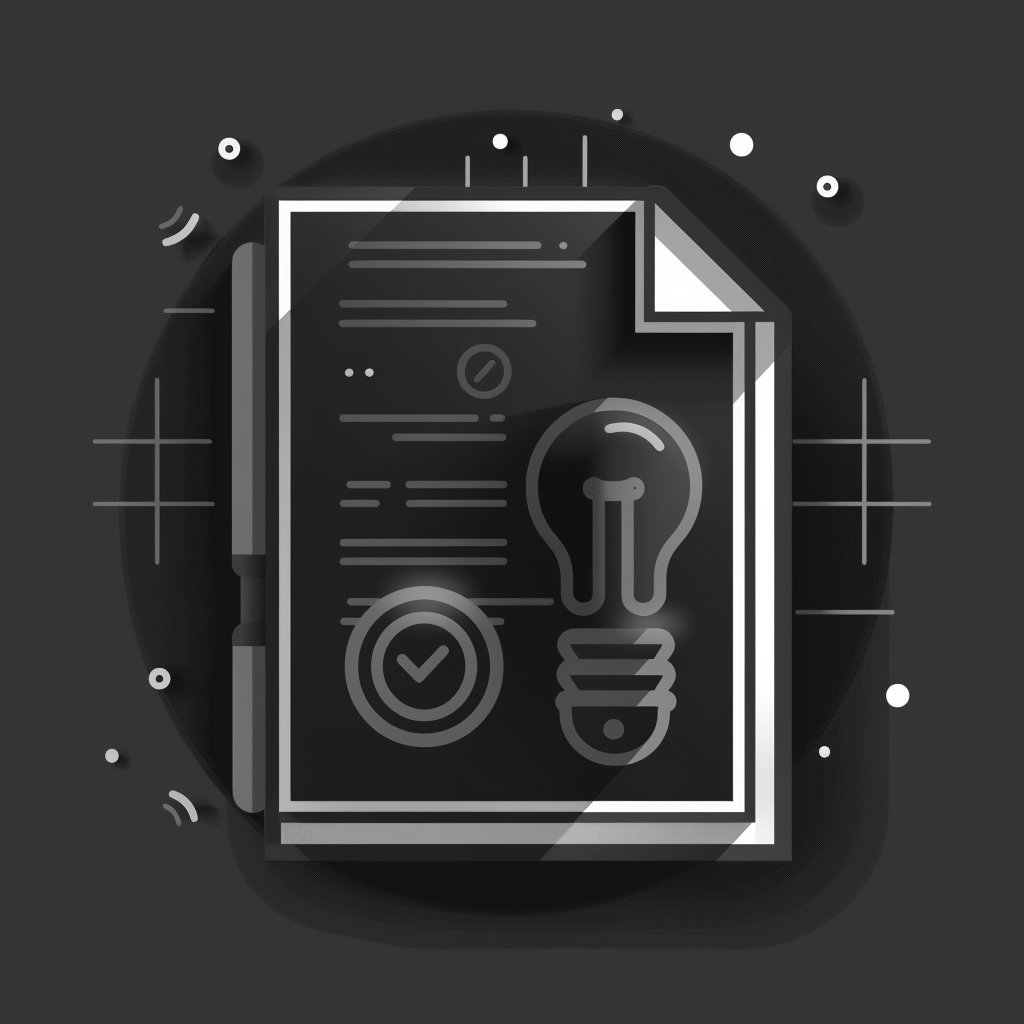
AIIF Masterclass: AI Capabilities and Evaluations
Last week, we dove into Gladstone AI’s report, and whether the recommendations align with other clear actions on AI from the U.S. government, such as the NIST framework, U.S. AISIC, and U.S. EO on AI.
This week, we’ll continue examining the Gladstone AI report by vetting the "Survey of AI R&D Trajectories" - the companion explainer for the Gladstone Action Plan.
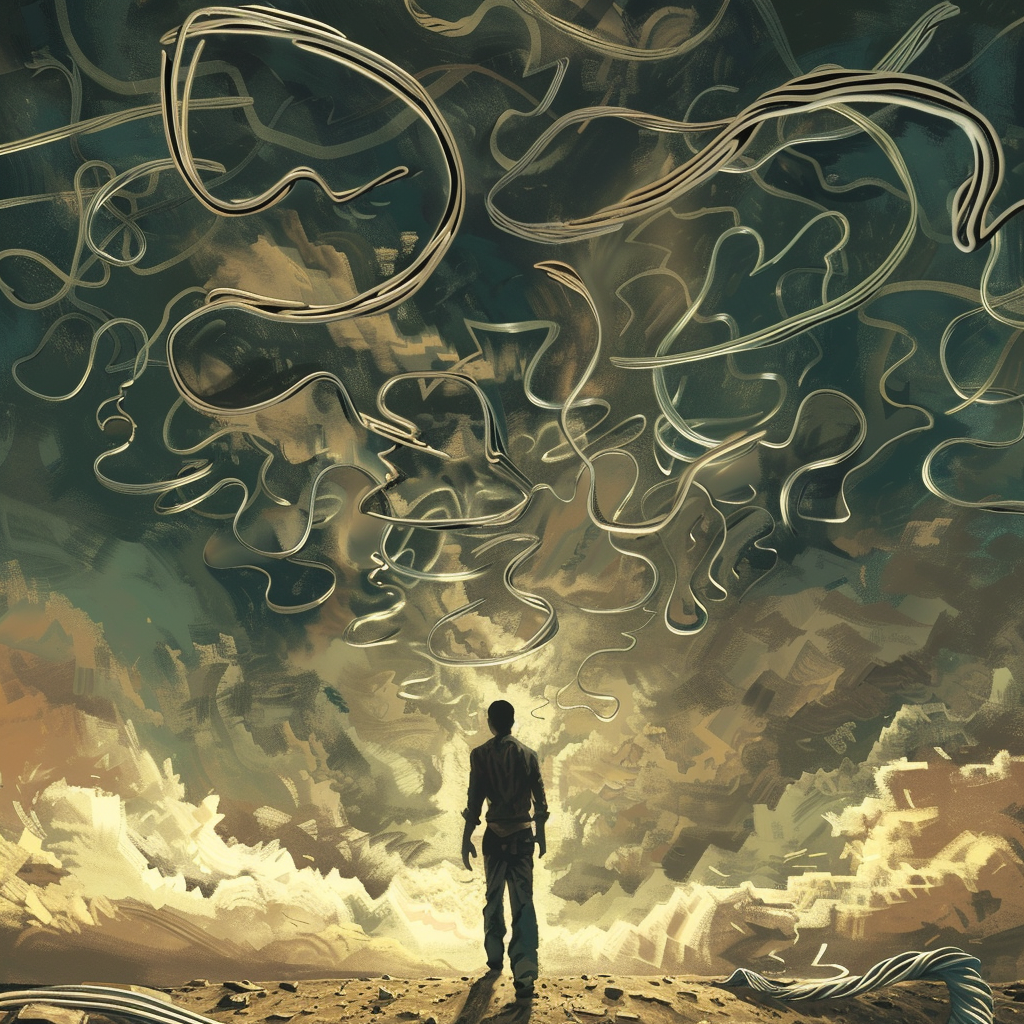
Guest Nathan Henry Presents: “A Hormetic Approach to the Value-Loading Problem: Preventing the Paperclip Apocalypse?”
This week, we’ve invited Nathan Henry to discuss his latest paper on the HALO algorithm (Hormetic ALignment via Opponent processes). HALO is designed to set healthy limits for repeatable AI behaviors and represents a cross-disciplinary approach to the value-loading problem.
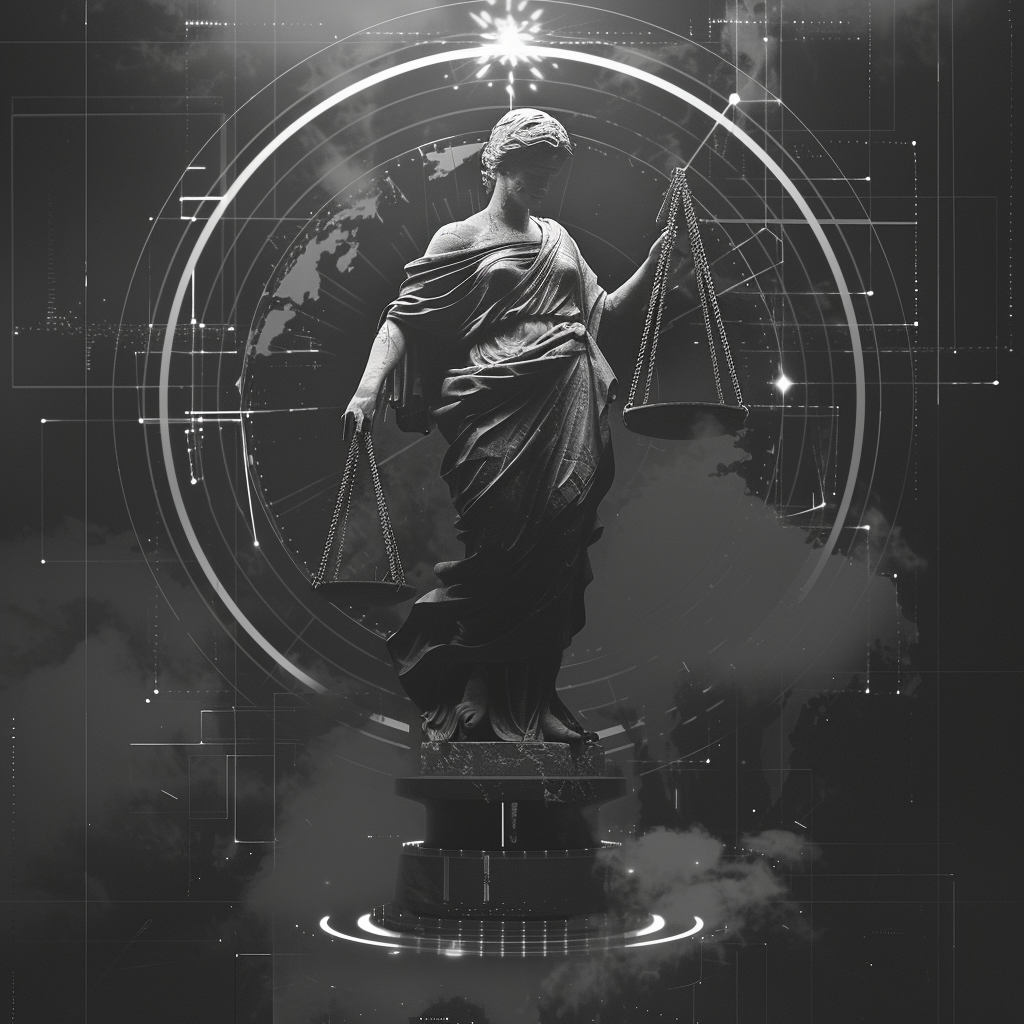
AIIF Masterclass: AI Rules
In 2023, the U.S. Department of State commissioned this report from Gladstone AI, an AI-related consultancy and current U.S. Artificial Intelligence Safety Institute Consortium (AISIC) member with ties to the U.S. military and apparent deep relationships in Silicon Valley. In February 2024, the report was completed and provided to the USDOS.

ML Benkyoukai: SORA
Whenever there’s an advance in charismatic domains like video generation, a media frenzy always accompanies. In the case of SORA, for once, the media frenzy may be justified; OpenAI’s new model marks a qualitative shift in what is possible when it comes to generating video and puts another strike in favour of brute scaling. Leaving aside the possible impacts on the stock video industry, is there anything interesting we can glean from their technical report? Any secret sauce other than more parameters → more performance?
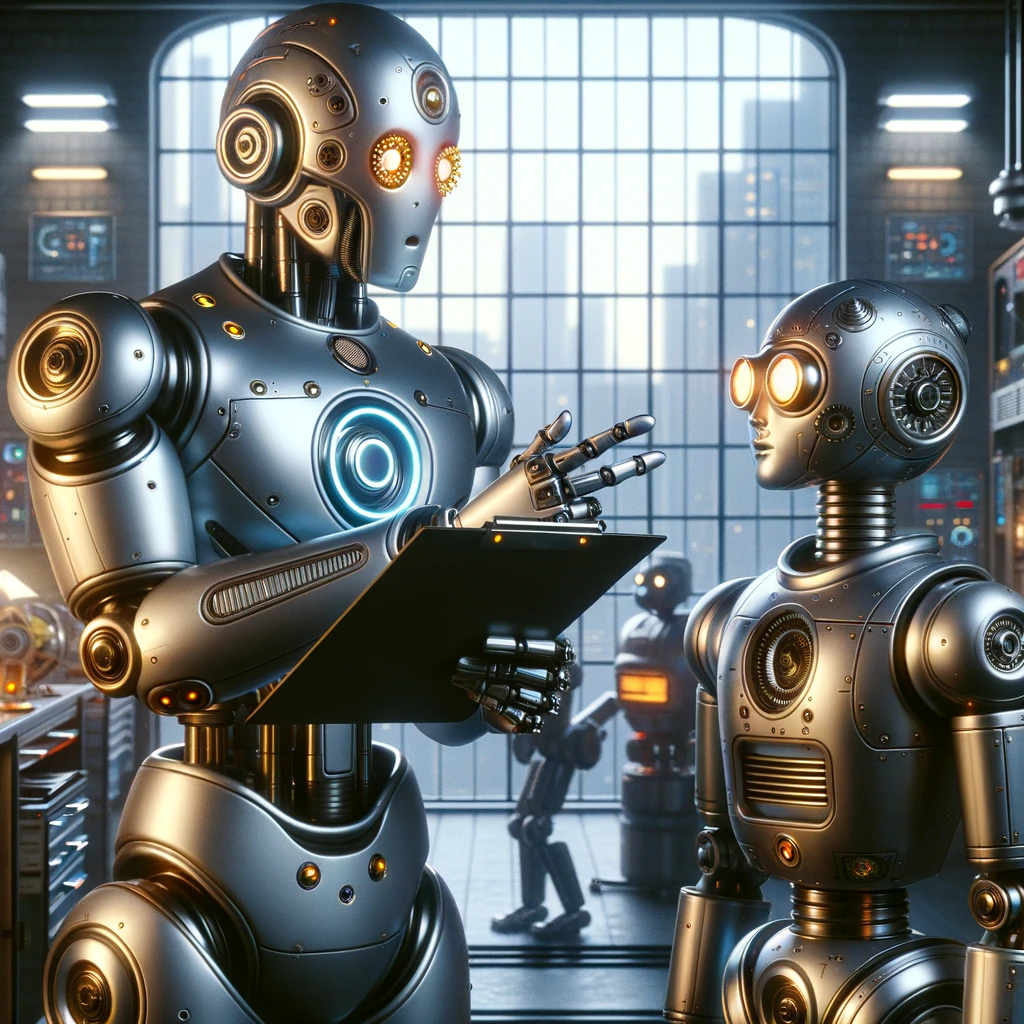
Reinforcement Learning from AI Feedback
A recent focus of ours has been reinforcement learning from human feedback, a technique for aligning AI (particularly large language models) to human preferences. A fundamental limitation of these approaches is the cost / quality tradeoff in the collection of the feedback from humans; most language models are trained on binary preference data (“which of these two continuations is better”) because humans can provide that feedback quickly and cheaply, not because it’s the optimal kind of data to train a language model on.
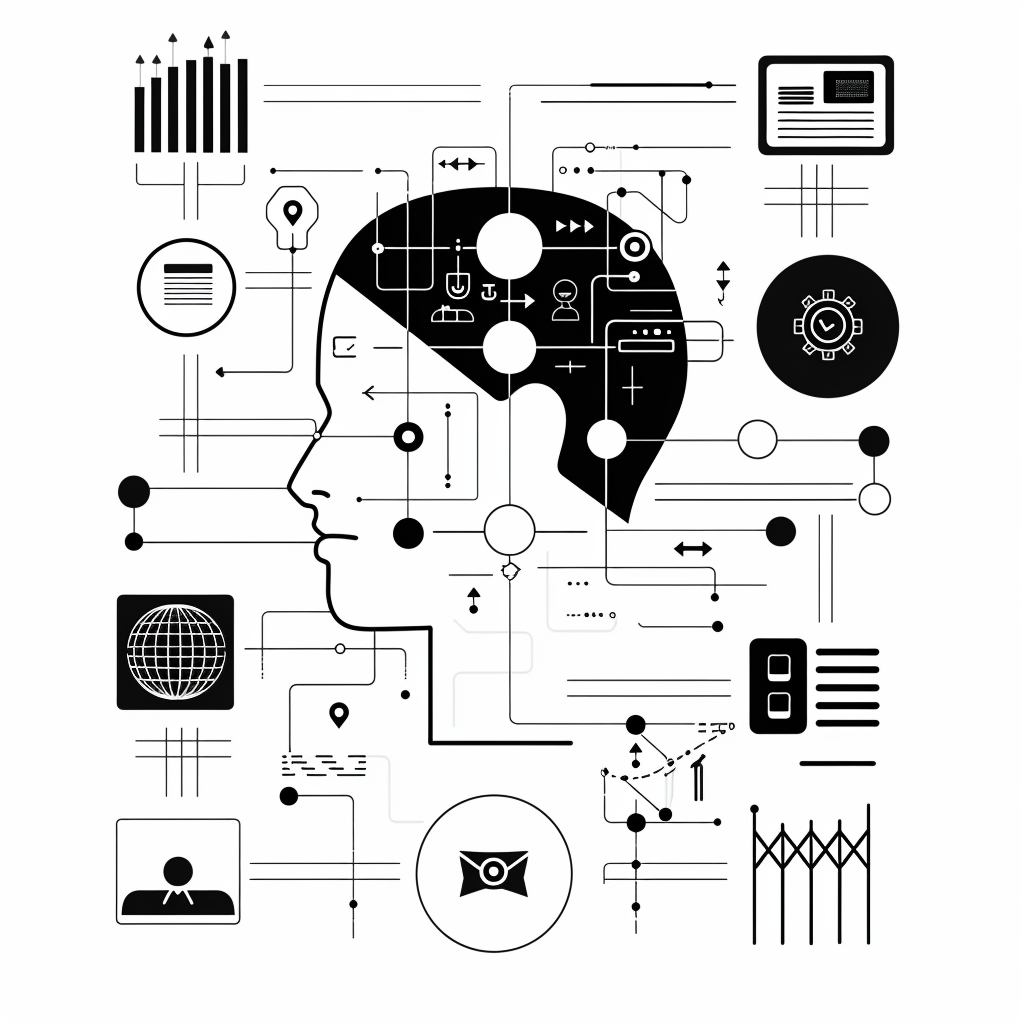
AIIF Weekly Masterclass: AI Use
Good mental models of generative AI systems are hard to find. Some people describe GPT as a “code interpreter for natural language”; others describe it as a surprisingly worldly five-year-old. A bad mental model can lead you to make bad inferences about what generative AI can do.
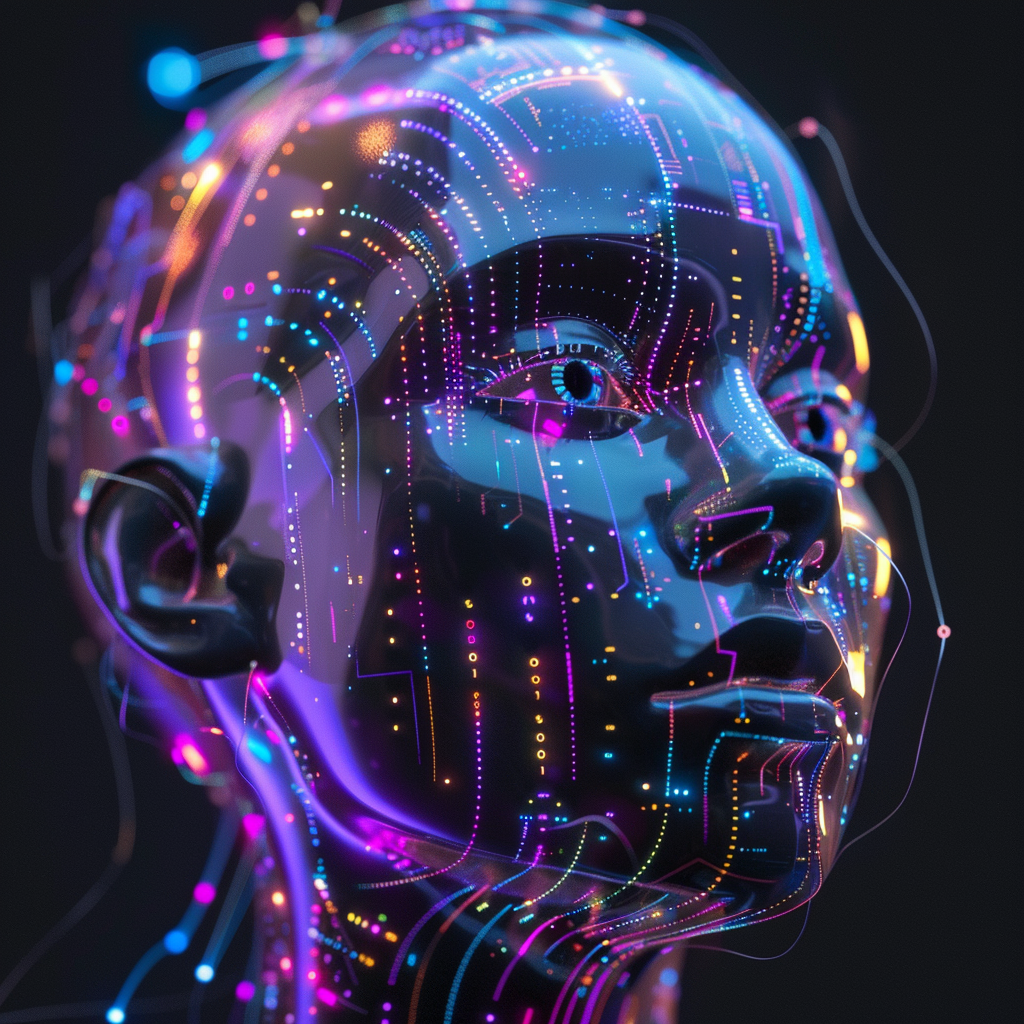
Fundamental Limitations of Reinforcement Learning from Human Feedback
A few weeks ago at the ML benkyoukai we talked about direct preference optimization, a successor to reinforcement learning from human feedback (RLHF) that addresses some of its technical limitations. But there are also more fundamental limitations with RLHF: are your evaluators aligned, either with each other or with humanity at large? Are their preferences worth learning? Are humans even capable of evaluating the performance of your model without being mistaken or misled?
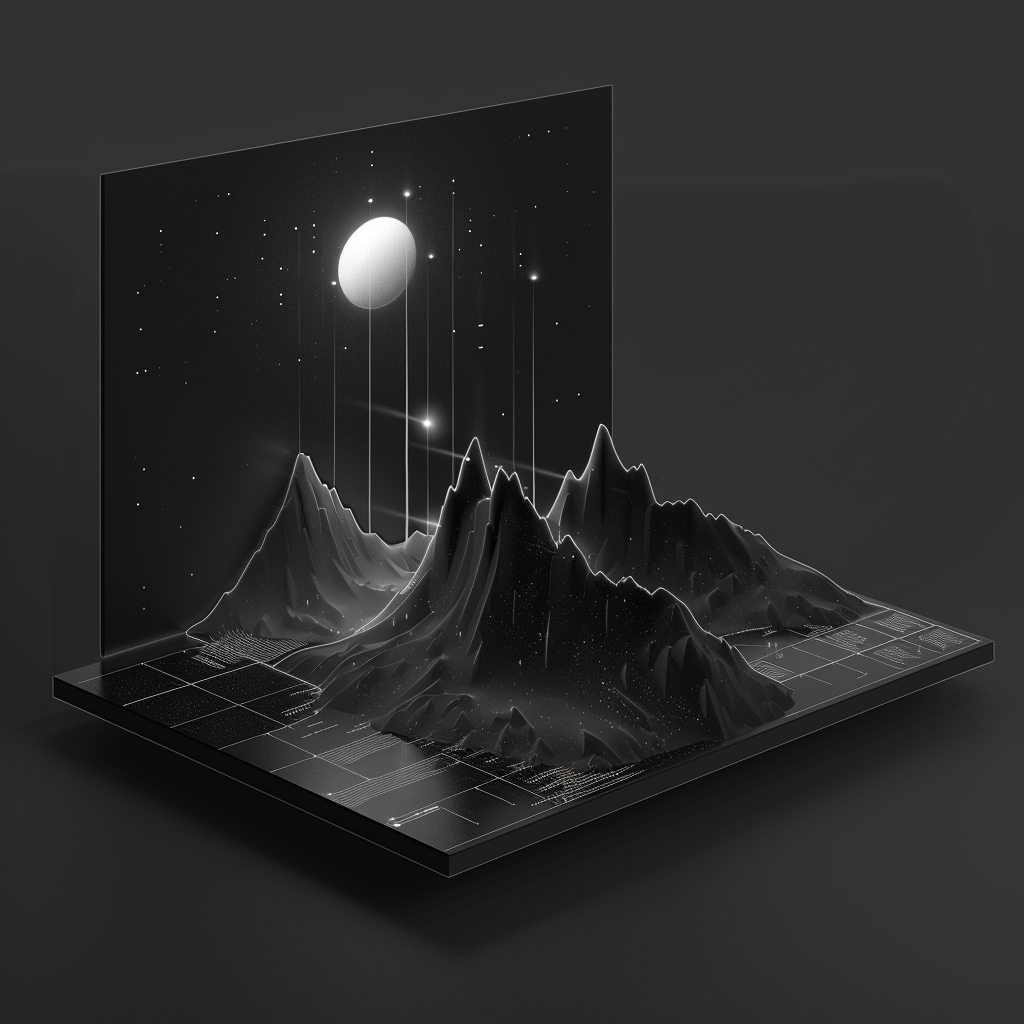
AIIF Weekly Masterclass: AI Landscapes
Welcome to the AI Industry Foundation’s first Weekly Masterclass.
The AIIF will be running Weekly Masterclasses to bring AI Executives up-to-speed. Our Masterclasses provide a structured distillation of technical and non-technical aspects of cutting-edge AI knowledge, preparing you to have conversations on the frontier of AI and to make business decisions based on those conversations.
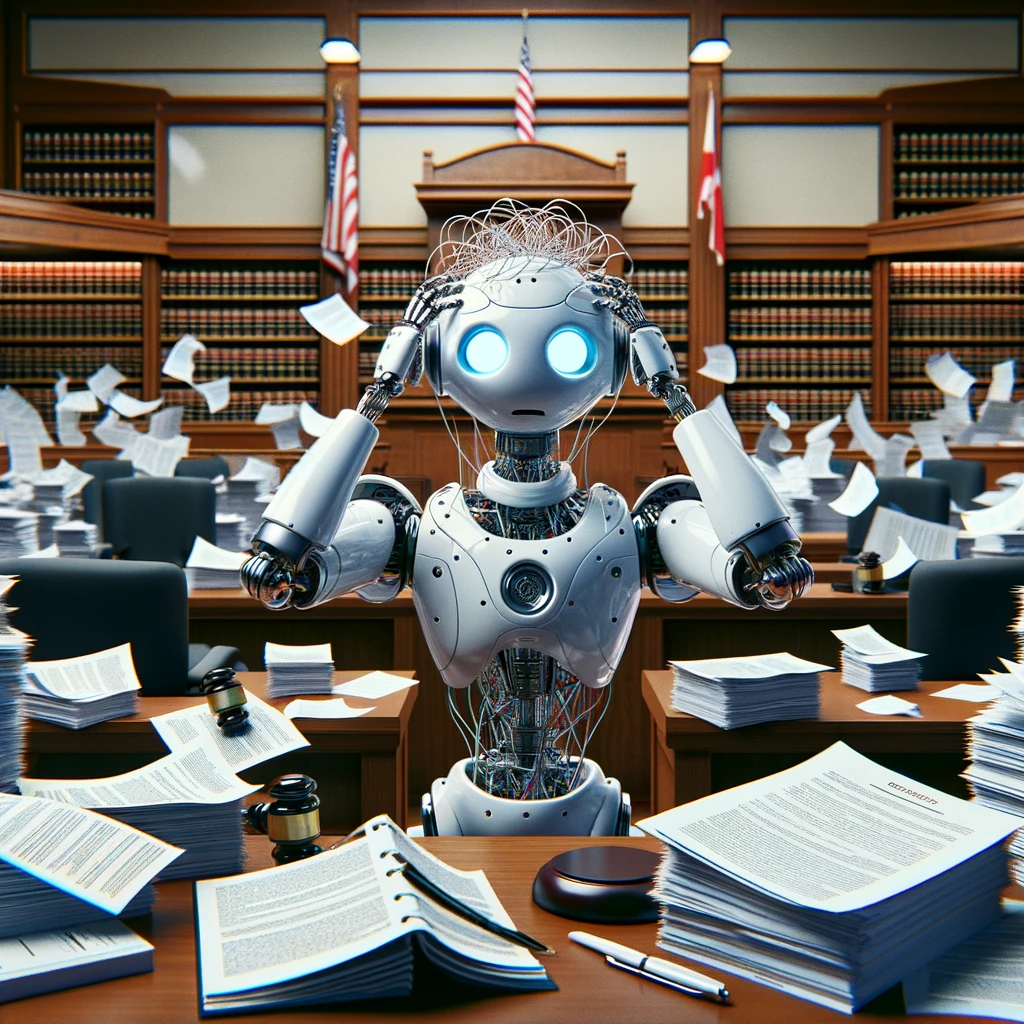
Elon Musk vs. Open AI
Last year saw Sam Altman ousted and then reinstated as CEO of Open AI amid concerns that it had drifted from its original non-profit mission. As events unfolded, we discussed how Open AI was formed and the various changes that have been made to the board over the years, including Sam Altman’s falling out with cofounder Elon Musk over the direction of the organization. This year, Elon returns to force things in court.
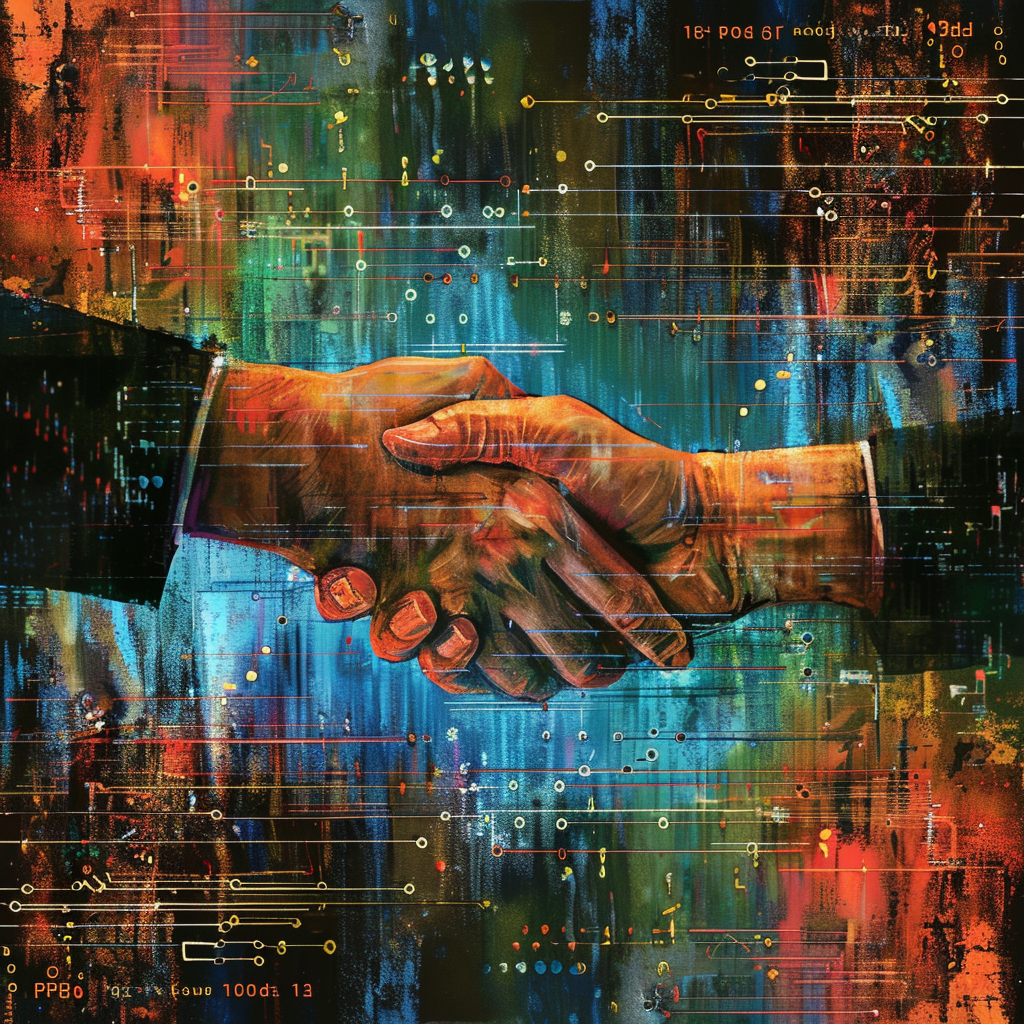
Meet the new boss of AI Safety: The U.S. AI Safety Institute Consortium (AISIC)
This week, Harold Godsoe will outline the American government’s blistering surge to get ahead on AI Safety, following up on the U.S. Executive Order 14110 on AI.
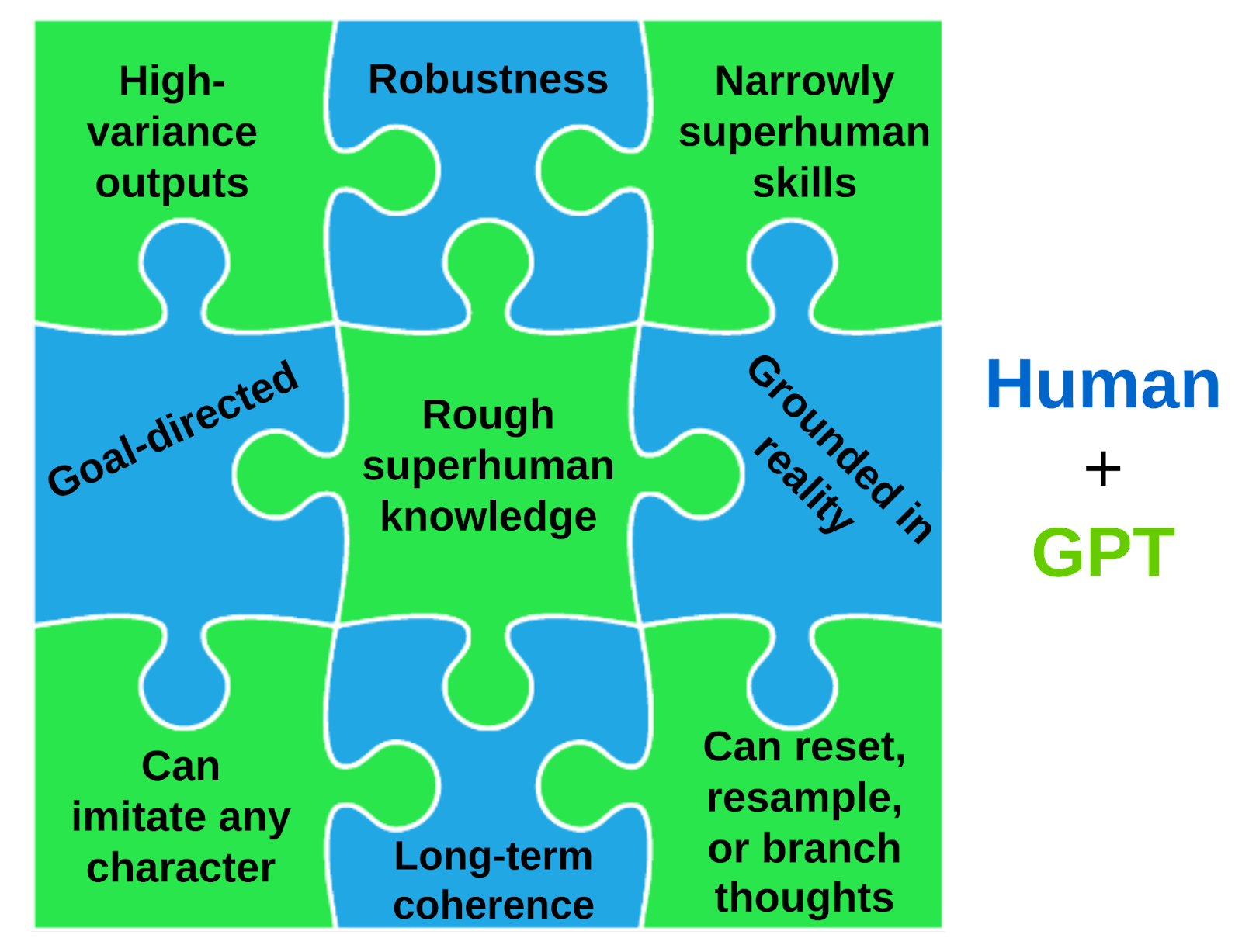
Cyborgism
Chat is the dominant way of interfacing with a large language model. You type some words into a chat box, and the model responds. Maybe you regenerate the response if you didn’t like the first one. Is that the best way to make use of a multimodal distribution over all of human language?
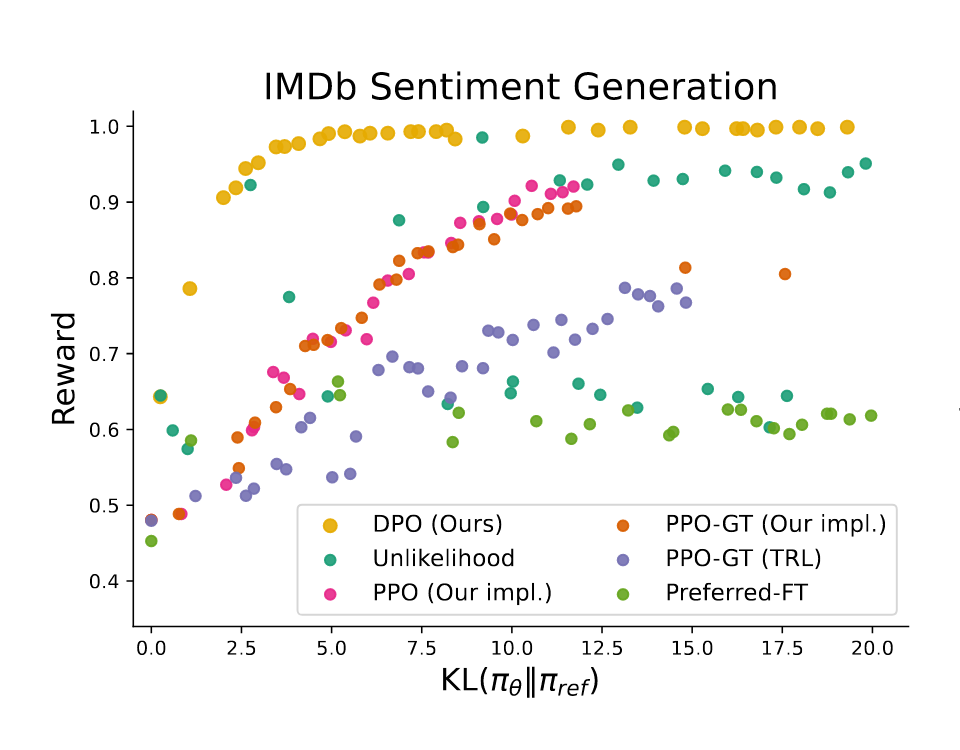
ML Benkyoukai: Direct Preference Optimization
[…]
Given the obvious importance of fine-tuning for getting good completions from large language models, it’s worth knowing the details. The most popular fine-tuning procedure, RLHF, is rather ad-hoc, and many people have suggested ways to improve it. One paper that has been getting a lot of hype recently is DPO (Direct Preference Optimization), which discards reinforcement learning in favour of just asking the model to produce preferred completions more and dispreferred completions less. They claim to get the same or better performance as RLHF, while being faster, easier to implement and more stable. This month we’ll use reviewing the DPO paper as an excuse to learn how RLHF works and survey the landscape of its competitors.
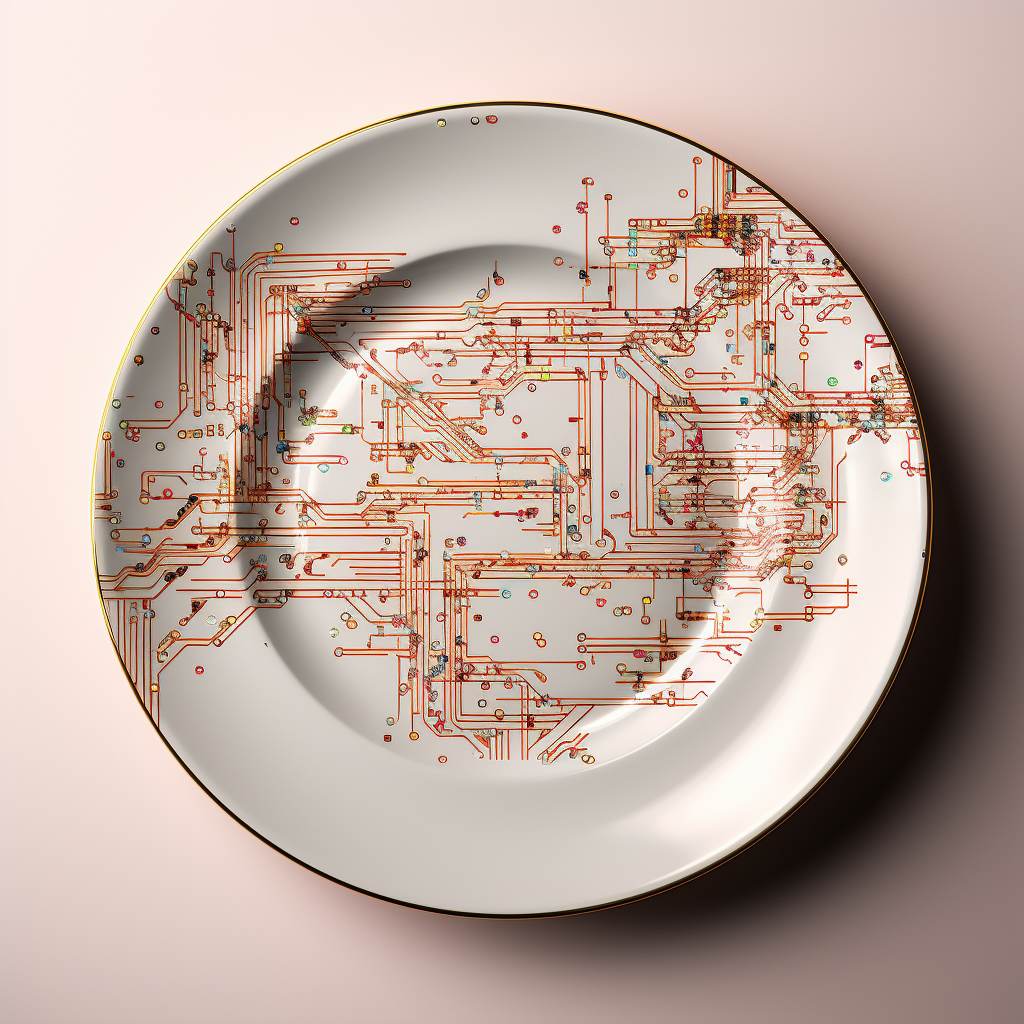
Guest Adelin Travers Presents: LeftoverLocals: Listening to LLM responses Through Leaked GPU Local Memory
As big labs become more aware of the safety (and business) implications of model weights leaking to bad actors, there has been renewed interest in cybersecurity and operational security in the safety community. Last December we discussed Anthropic’s Responsible Scaling Policy, which included cybersec / opsec recommendations we described as “things smart people who don’t know about cybersec / opsec would recommend”. Perhaps we should engage more closely with the cybersecurity community!
To that end, this week we have invited Adelin Travers as a guest speaker. Adelin works with the team at Trail of Bits which recently uncovered an important vulnerability (LeftOverLocals) that impacts GPUs used in ML systems. Their original blog post was also covered by Wired Magazine just a few weeks ago, on January 16th.
During the session, Adelin will give an overview of the intricate tech stack behind modern machine learning, making clear the extent of the attack surface. He’ll explain the LeftOverLocals vulnerability, and what implications it has for users and developers of AI services. We’ll discuss where LeftOverLocals stands in the general landscape of vulnerabilities, and the appropriate level of worry. We’ll also discuss what practices organizations can adopt to insulate themselves against LeftOverLocals and other vulnerabilities of its kind.
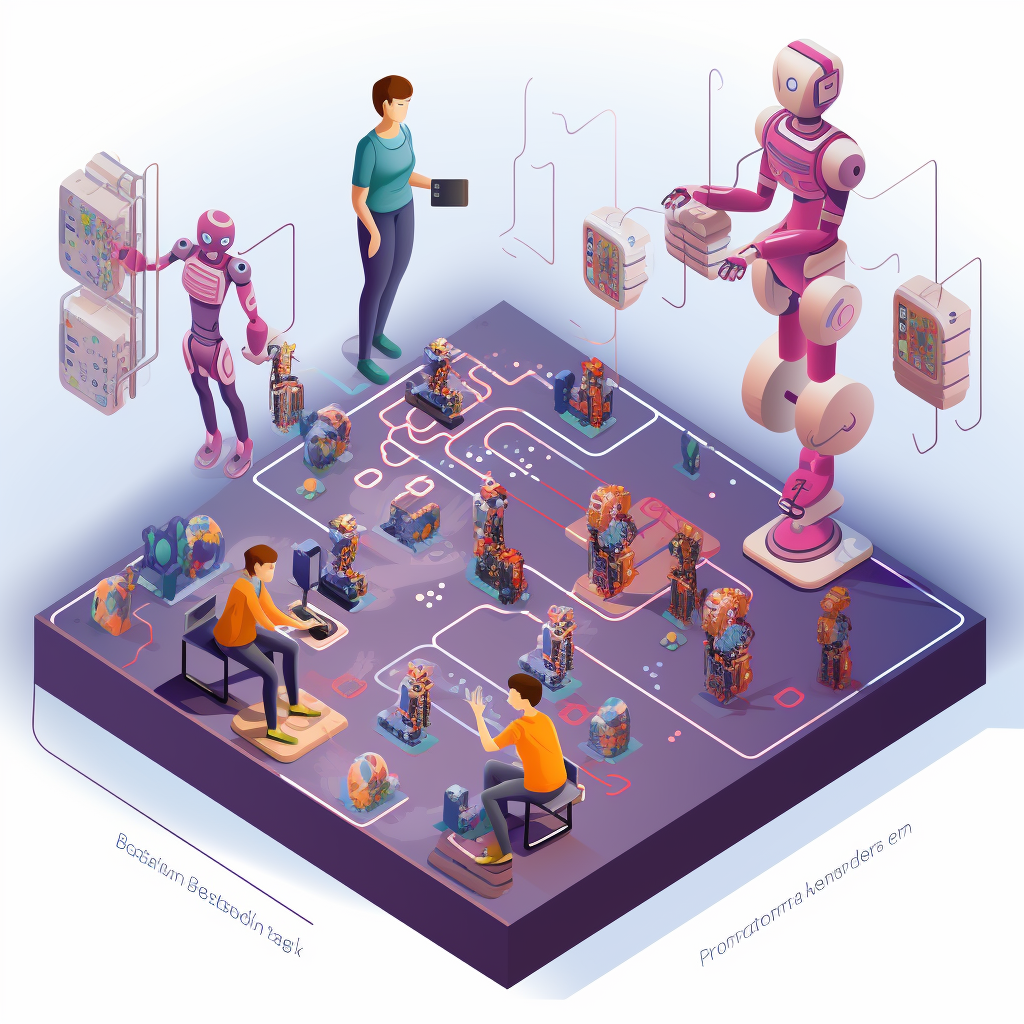
Why Agent Foundations?
Alignment is hard. Research directly aimed at the “big problems” (incorrigiblity, misaligned convergent instrumental subgoals, etc) seems disconnected from the practicalities of how AI research gets done. In the early days of alignment research, much ink was spilled over how to correctly specify utility functions for reinforcement learning agents. Large language models, “the closest thing we have to AGI”, came from a totally different paradigm, making the effort seem silly in retrospect.
Given that framing, why do many alignment researchers continue to pursue “foundational” topics, instead of more tractable approaches like mechanistic interpretability, model evaluations or compute governance? In his article “Why Agent Foundations?” John Wentworth attempts to answer this question; we’ll use the article as a jumping off point to survey the work being done in agent foundations and ask whether too much or too little agent foundations research is being done given the rest of the safety research landscape.
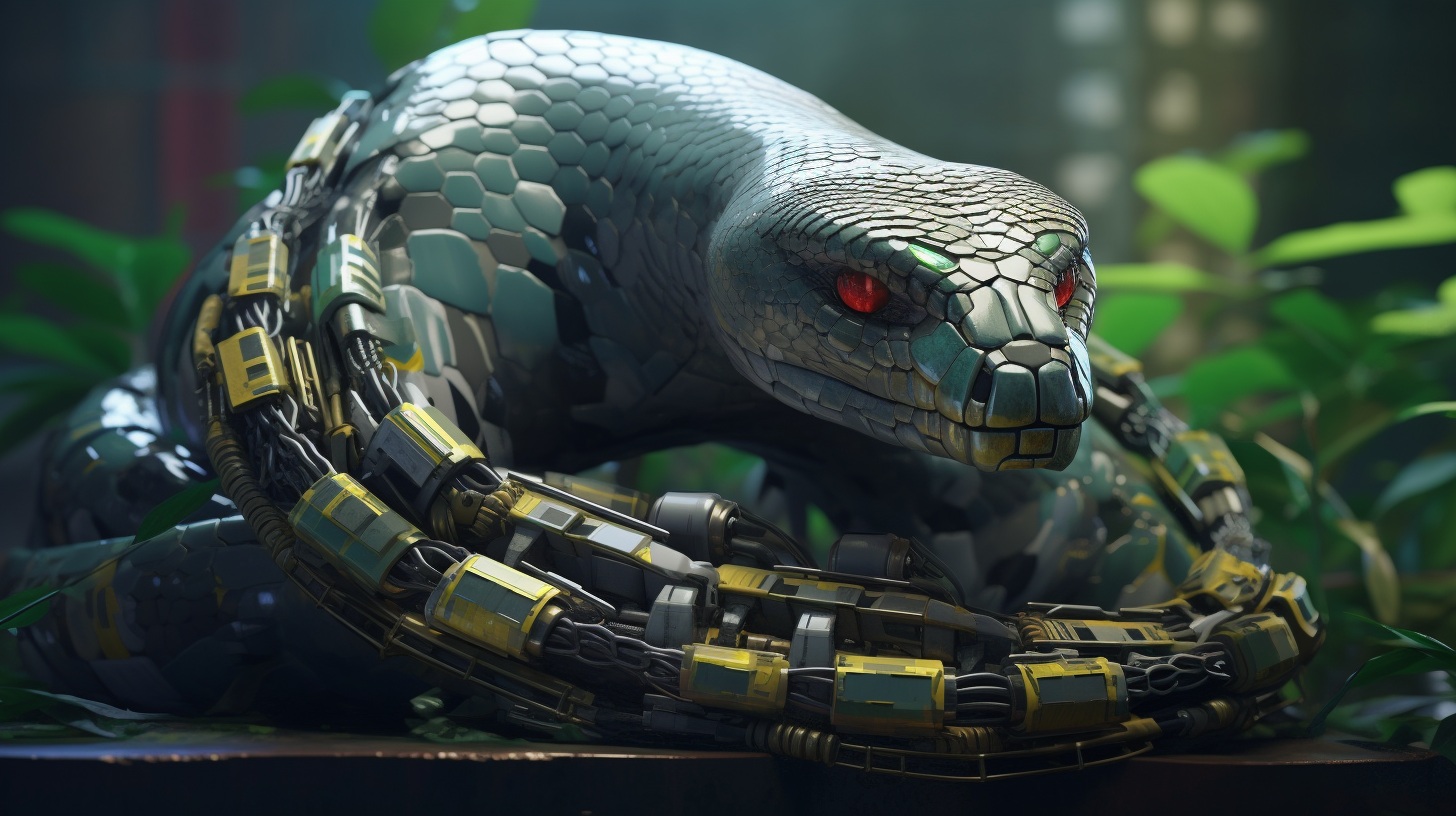
ML Benkyoukai: Mamba
Are we at the precipice of a foundation model architectural revolution?
In the majority of our study groups, we’ve looked almost exclusively at large language models, often billed as “the closest thing we have to artificial general intelligence”. These large language models all use the transformer architecture. Transformers have proved very powerful, but are hamstrung by their poor scaling with sequence length / context window size. In the past few years there have been many attempts to modify the transformer architecture to scale better without harming performance, to varying degrees of effectiveness (Performer, Reformer, Random Feature Attention, Transformers are RNNs, LongNet, to name a few). But what if instead of modifying the transformer to be more efficient, we could replace it with an architecture designed for efficiency from the ground-up?
The much hyped Mamba, released in December 2023 by Gu and Dao, promises to be just such an architecture. Mamba (so called because it uses a Selective Structured State Space Sequence-model or SSSSS🐍) replaces the attention mechanism, which scales quadratically with sequence length, with a structured state space sequence model that is linear in sequence length during training and constant (!) in sequence length when deployed. Structured state space sequence models are not Mamba’s invention; their trick is to drop the linear time invariance constraint adopted by other authors, then optimize hard for training and inference on GPUs to make dropping that constraint feasible. In evaluations, the Mamba architecture achieves state-of-the-art performance on various modalities such as language, audio, and genomics, matching transformers twice its size.
What should we make of this? Will the selective structured state space sequence model come to replace the transformer, as the transformer replaced the convolutional neural network before it? How does the SSSSS compare to previous recurrent architectures like the long short-term memory network or the neural Turing machine? Is a 2x improvement in performance even a big deal when you can 100x your performance with <1% increase in training compute using reinforcement learning from human feedback?
All these questions and more will be answered in the first session of AI Safety Tokyo’s machine learning benkyoukai. We will be going much deeper into the details than our usual benkyoukai. Expect to hear terms like activation function, skip connection and convolution thrown around without explanation. Unlike our regular benkyoukai, we’ll be expecting our attendees to have read the paper beforehand.
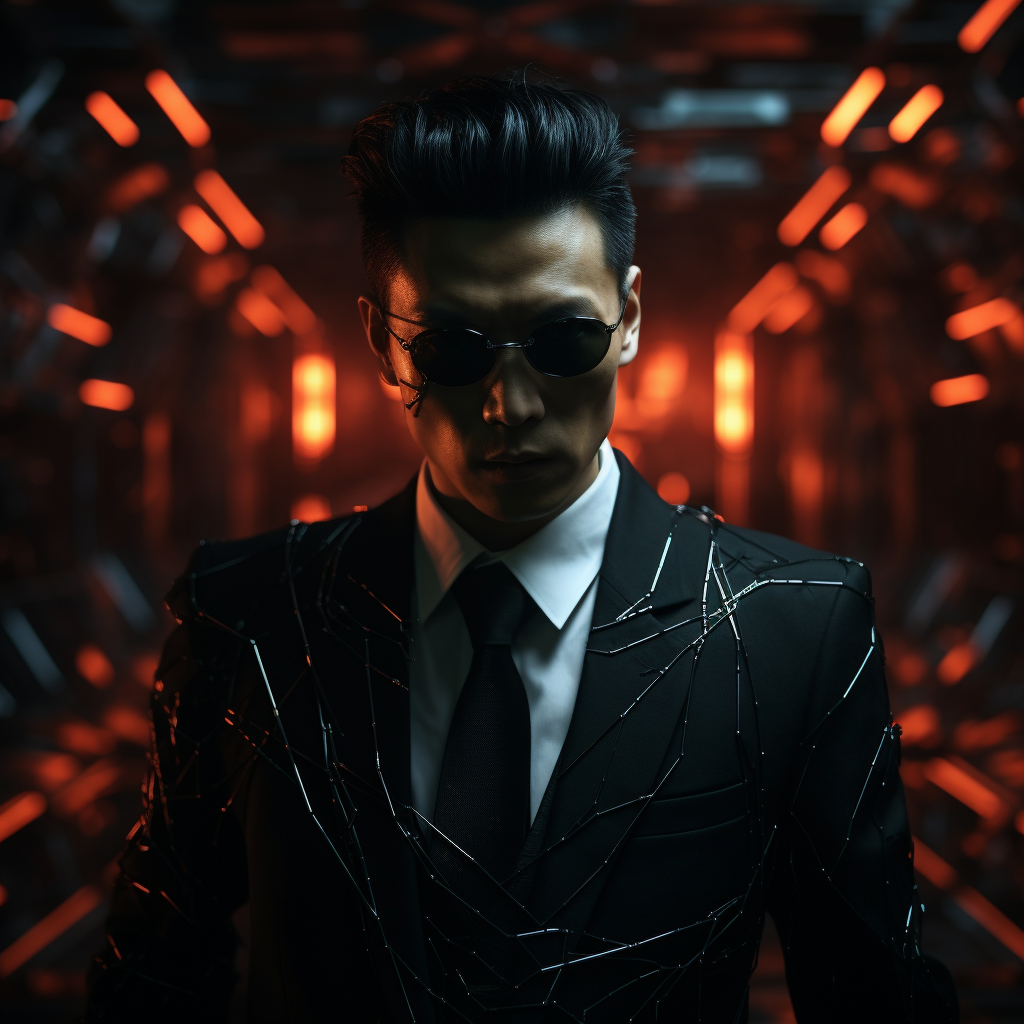
AI Sleeper Agents
Most of us are familiar with the idea of “sleeper agents” from cheesy action movies from the 80’s and 90’s. For those of you not familiar: A "sleeper agent" refers to an individual who is covertly planted in a target area or organization, with the purpose of remaining inconspicuous until “activated” to carry out a specific mission or task. These agents are often trained to blend into their surroundings and may live seemingly normal lives until they receive instructions to engage in espionage or other covert activities.
Much as spies worry about sleeper agents in their midst, alignment researchers worry about deceptively aligned AIs. If an AI were to spontaneously develop deceptive tendencies during training, would our most popular alignment techniques (reinforcement learning, supervised fine-tuning and adversarial training) be enough to keep it in check? In a fresh paper released less than a week ago, Hubinger et al. perform experiments with Anthropic’s Claude and find that the answer is no.
Some people find this interesting and important, and others not so much. Blaine is still deciding. This week we’ll explore LLM sleeper agent deception: are we safe?
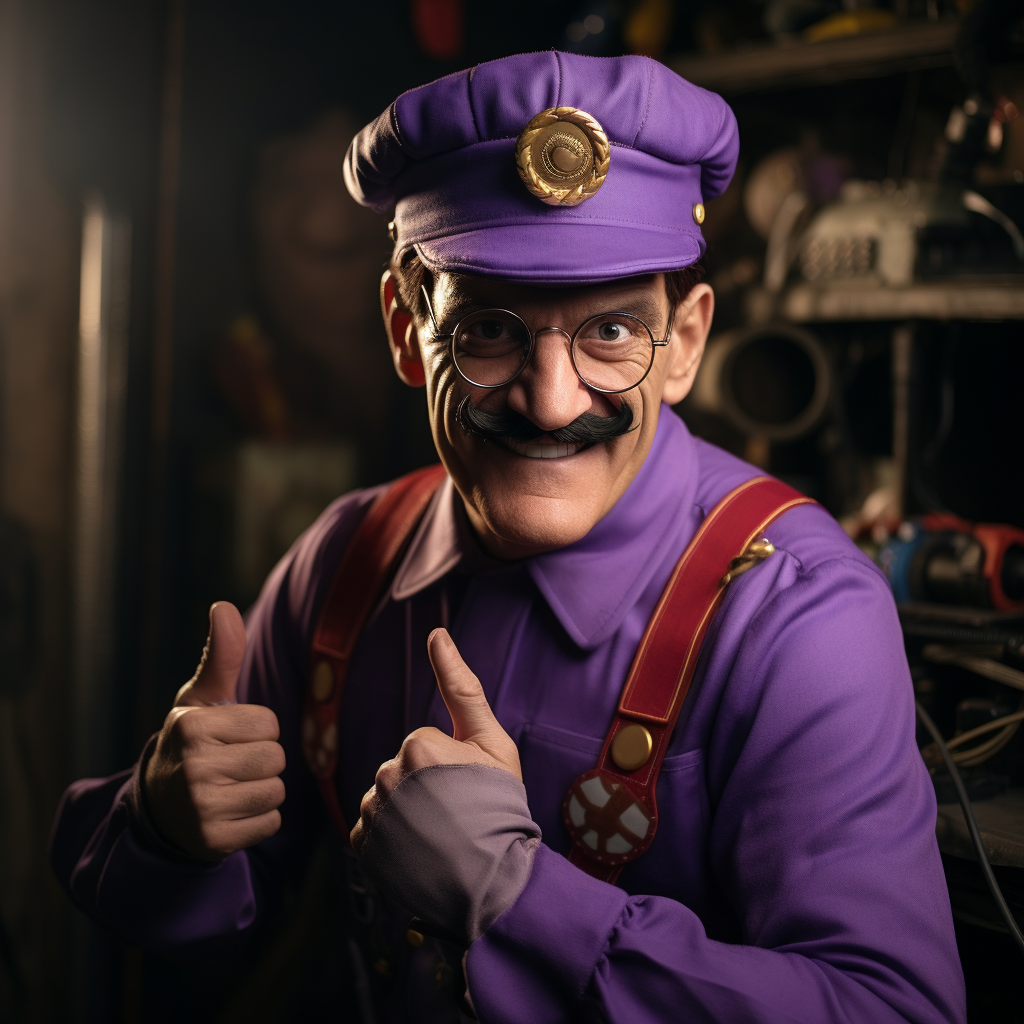
The Waluigi Effect
Large language models are the closest thing we have to artificial general intelligence, and their nature is still poorly understood. Last week we asked whether large language models were able to solve hard compositional tasks (and found that they couldn’t, even after being trained exhaustively on easy compositional tasks). That paper took a quantitive stance; this week we’ll follow up with The Waluigi Effect, which takes a qualitative stance.
The Waluigi Effect, following on from Janus’ Simulators, investigates the “semiotics” of large language models. It centres its exploration around a claim about the geometry of simulacra: that it is harder to define a trait than it is to specify whether or not a simulacrum has the trait. This, coupled with the tendency of stories to have narrative twists in which a character pretending to be good reveals themself to be evil, primes large language models to do the opposite of what you ask them. If evil is the opposite of good, you can go from good to evil by flipping a single bit!
The Waluigi Effect was published almost a year ago, in March 2023. Does it still hold up 10 months on? What can the narrative structure or semiotics of the user / AI assistant dialogue genre tell us about the tendencies of modern digital assistants? What third question can I put at the end of this paragraph that will complete the rule-of-three pattern you expected at the start?
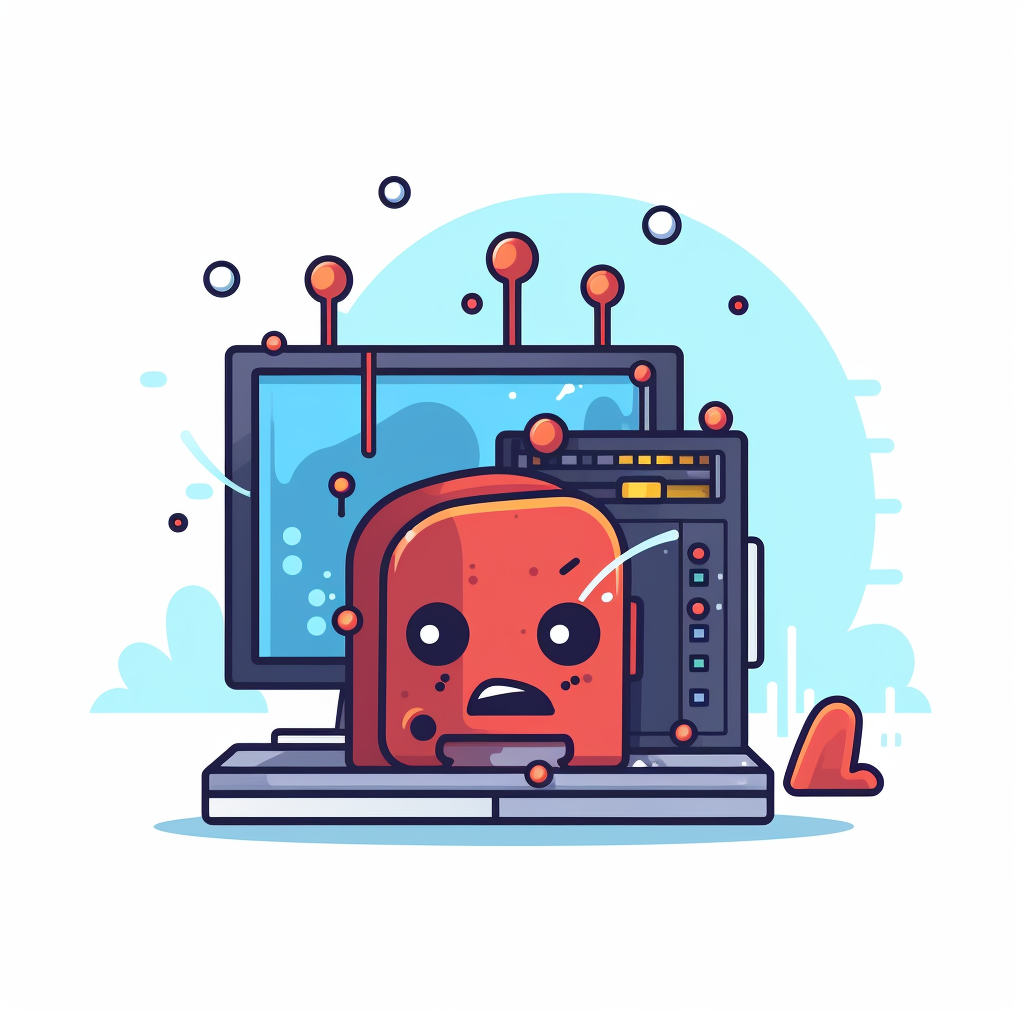
Limits of Transformers on Compositionality
Much ink has been spilled on whether large language models “actually learn”, instead of “just memorizing patterns”. Is Chat-GPT a stochastic parrot, or a human simulator? How would we tell the difference?
In a recent paper, Dziri et al explore how large language models solve compositional tasks—tasks that need to be broken down into smaller tasks, which in turn need to be broken down into smaller tasks in order to be solved. They suggest that LLMs are indeed stochastic parrots; they solve these tasks through memorization, becoming increasing prone to error the more unusual the example or the more complicated the task: “models are able to correctly perform single-step reasoning, potentially due to memorizing such single-step operations during training, but fail to plan and compose several of these steps for an overall correct reasoning”. They find this persists even if they explicitly fine tune the models on task specific data, train the model to use a scratchpad to track its work in progress, or train the model far beyond overfitting (“grokking”).
In our next session Blaine will start the New Year off reviewing the main takeaways from this study, and relating them back to AI safety. How should this affect our view on AGI timelines? Is this a limitation of the transformer architecture that can be easily overcome? What does this mean for people who want to use large language models for mundane utility? All this and more in the first benkyoukai of 2024.
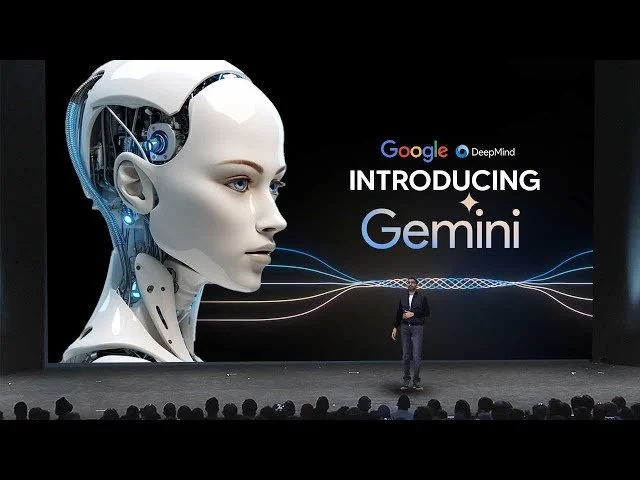
The Gemini Report
Or, “Blaine read the Gemini Report, so you don’t have to.”
On December 6th, Google announced it’s new and “most powerful LLM model ever”: Gemini. Excitement and hype followed, then waves of scrutiny. Even given Google’s cherry-picking demos, it seems that Gemini Ultra is better than GPT-4 on almost all benchmarks. That and its native multimodal capabilities make Gemini a genuine improvement on the state of the art, and worthy of our attention.
This week, Blaine will give a close reading of the Gemini report, with a healthy degree of skepticism. What technical details can we glean from Google Deepmind’s vague descriptions? Will a 67% to 74% improvement on benchmarks be a noticeable to users? Is the Gemini release healthy competition, or irresponsible accelerationism? Should the working professional prepare to cancel their GPT-4 subscription and jump ship? Let’s find out.
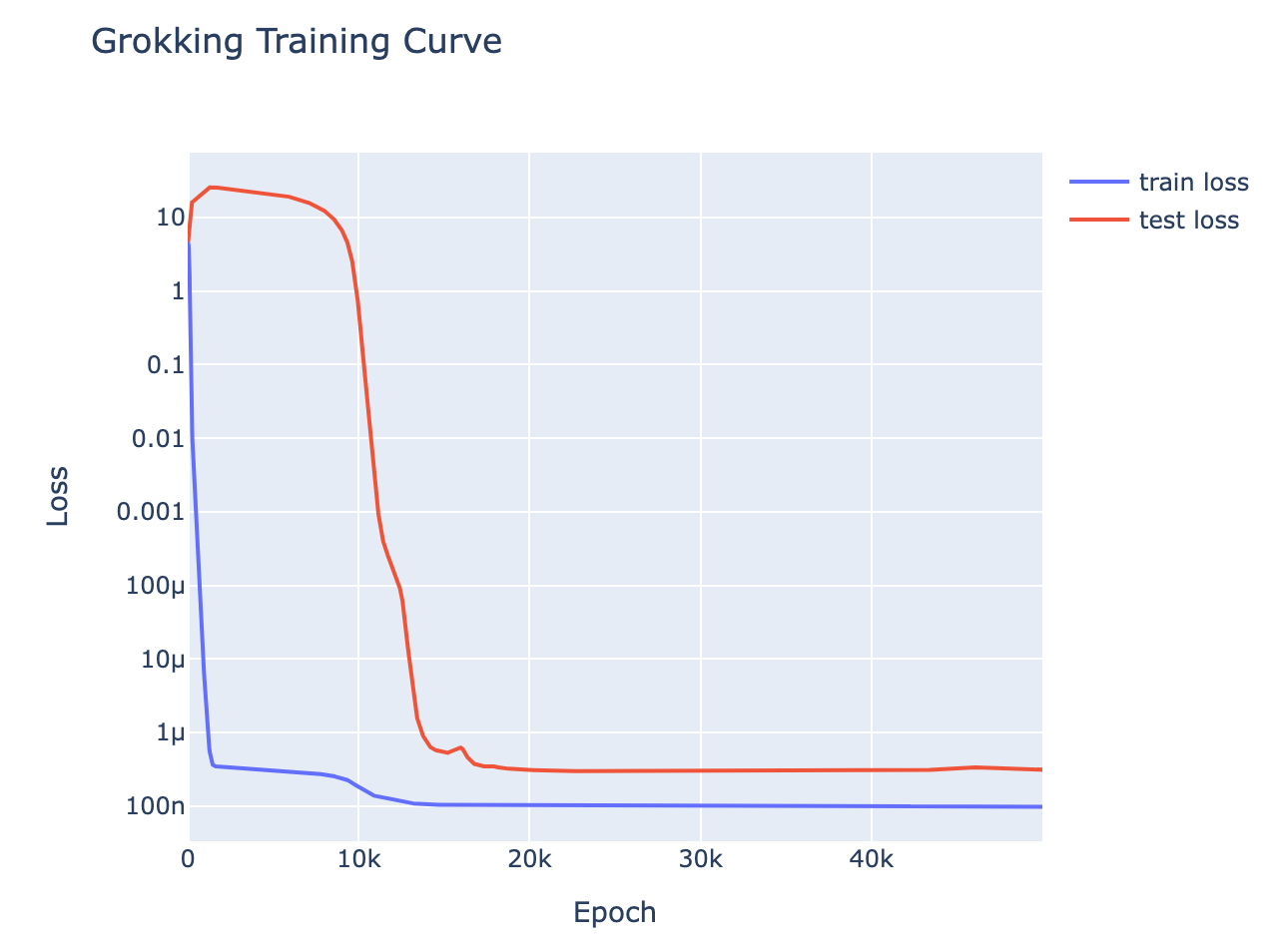
Progress Measures for Grokking via Mechanistic Interpretability
Grokking: what is it, and why should we care?
In 2022, OpenAI researchers discovered something: models trained on small algorithmic tasks (like modular addition) will initially memorise the training data. Then “after a long time”, will suddenly learn to generalize to unseen data. From the linked Alignment Forum post: “One of the core claims of mechanistic interpretability is that neural networks can be understood, that rather than being mysterious black boxes they learn interpretable algorithms which can be reverse engineered and comprehended. This work serves as a proof of concept of that, and that reverse engineering models is key to understanding them.”
This week, we’ll recap some background on grokking, double descent and related phenomena. Then we’ll analyze the claims of Nanda et al., and inspect the links between grokking and phase changes. If we have time, we’ll relate the phase changes to the developmental interpretability agenda we’ve covered in previous session, examining some recent work calculating the RLCT for phases in modular addition.
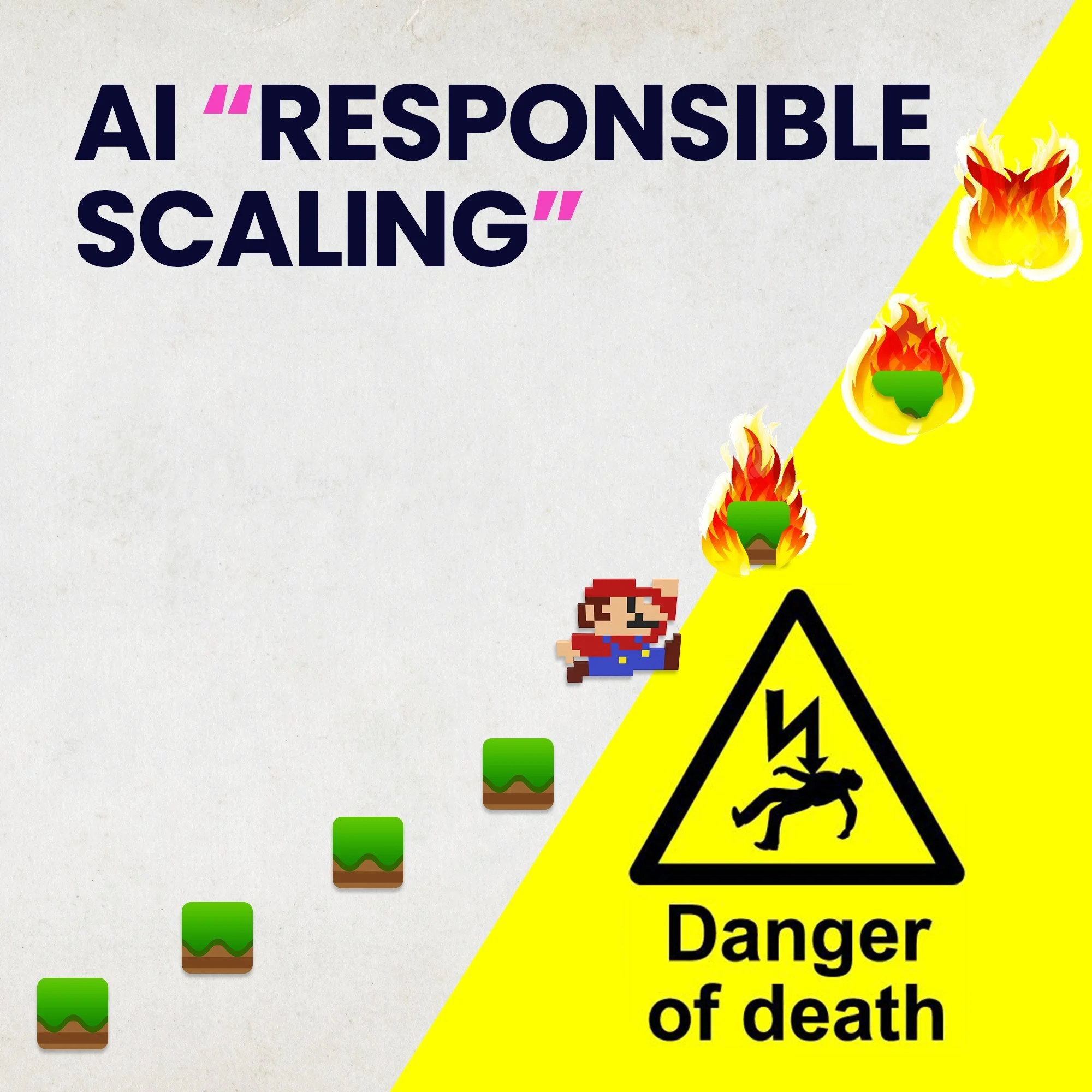
Anthropic’s Responsible Scaling Policy
In September, Anthropic published their Responsible Scaling Policy outlining the protocols they will be adopting to help manage “increasingly capable AI Systems.”
There are rumours and whispers about OpenAI supposedly having a secret AGI they’ve been hiding from the public; a salient take on responsible policies to prevent such a thing from happening seems prudent.
We’ll take a critical look at the AI Safety Levels (modeled after the US government’s biosafety level standards), discuss whether these safety standards are enough, and explore the possible industry impacts as other companies are expected to fall in line.

The OpenAI Debacle: what does this mean for the future of AGI development?
As many of you know, over the past week there’s been nearly hourly updates on OpenAI’s recent “15-minute firing” of Sam Altman and the subsequent firestorm that lit up OpenAI and the board members responsible for blindsiding both the public and OpenAI’s investors.
We will examine how OpenAI’s Board impacted the future of OpenAI and AGI development. Most notably, Helen Toner who published a very recent academic paper, criticizing OpenAI’s “frantic corner-cutting” in the release of ChatGPT (as opposed to praising Anthropic’s more “safe” approach of a delayed release of it’s GPT-4 chatbot, Claude).
Sensationalism and conspiracy theories aside, in this session we’ll examine OpenAI’s original board charter, review the facts of what happened, the makeup of OpenAI’s new Board, and speculate on how the week’s events will impact the future of AGI development.
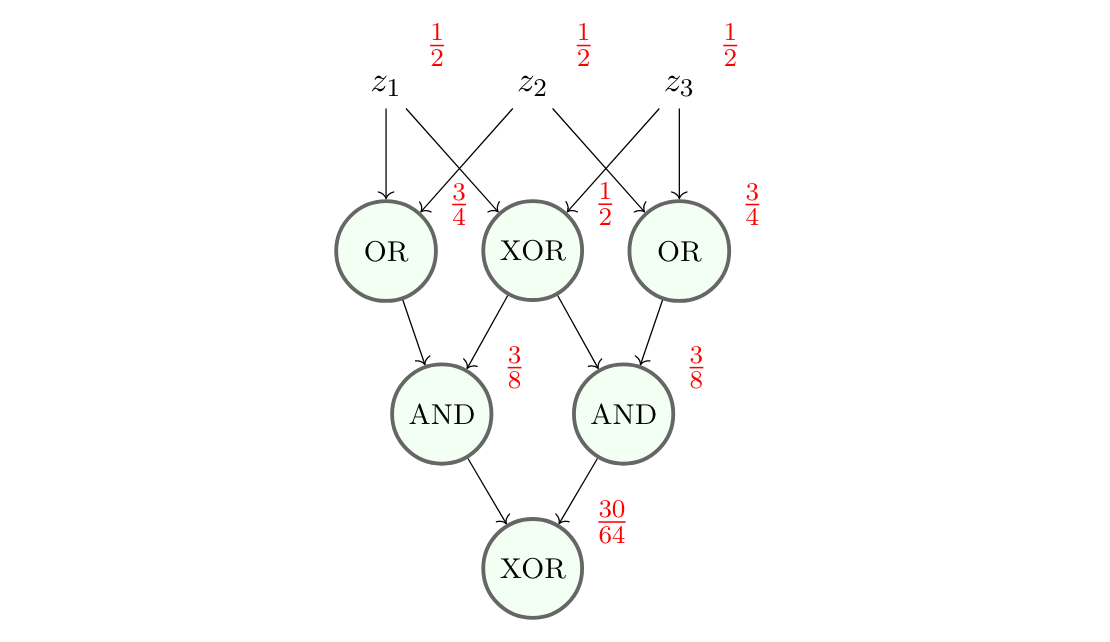
Formalizing the Presumption of Independence
Paul Christiano is a prominent figure in AI safety that we have so far overlooked. His institute, the Alignment Research Center (ARC), is well known for its work on model evaluations and eliciting latent knowledge. In his most recent paper with Eric Neyman and Mark Xu, Christiano proposes a new research direction: formalizing an obscure kind of defeasible reasoning.
This week, Blaine Rogers will trace the connection between defeasible reasoning and AI safety. In doing so, he’ll relate the aforementioned to Anthropic's work on transformer circuits, Redwood Research's work on causal scrubbing, MIRI's work on logical uncertainty, and (if time allows) ELK—ARC's other research direction.

What is Bayesian Machine Learning?
Last month, while discussing Toy Models of Superposition, we briefly touched upon phase transitions in the Bayesian posterior (specifically the MCMC posterior sampling).
This week Blaine will cover the basics of Bayesian machine learning, including: Bayesian probability from first principles, Bayesianism / frequentism approaches, what Bayesianism means for neural networks, and the current meta of tools & techniques for Bayesian ML.

Briefing: The Executive Order on the Safe, Secure, and Trustworthy Development and Use of Artificial Intelligence
On October 30th, 2023, US President Biden issued a new Executive Order on the Safe, Secure, and Trustworthy Development and Use of Artificial Intelligence. Similarly, the UK hosted an “AI Safety” summit (alternatively called the “doom summit” by British media) with two main aims:
to consider the risks of AI, especially at the frontier of development,
to discuss how they can be mitigated through internationally coordinated action.
In this session, Harold Godsoe will give a summarized briefing of the Executive Order, explore the legal implications, and expound on the future of international AI safety and alignment regulations (through a legal lens).
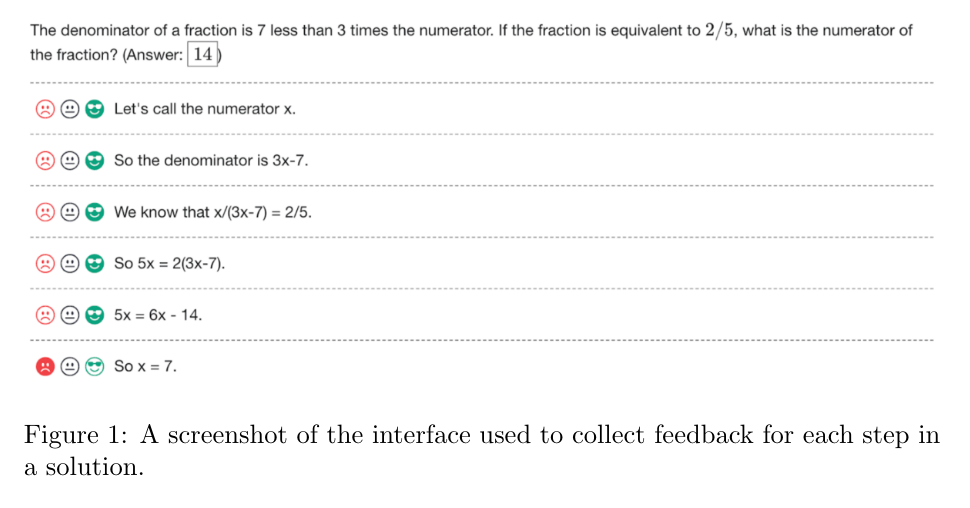
Process Supervision
This week, we’ll explore a recent work from OpenAI: the lab-to-watch with the biggest and best models. It's pretty on-the-mark for their proposed Superalignment approach.
At our session on Representation Engineering, we had an argument about symbols and referents—when an AI says "I am a corrigible AI and I will let you turn me off," what does that mean? Is it being truthful? This paper, mostly targeted at capabilities, aims to get models to produce 'aligned' chain-of-thought reasoning. Is writing “aligned words” equivalent to being aligned? Are we who we pretend to be?